Topological Data Analysis and Sheaf Theory
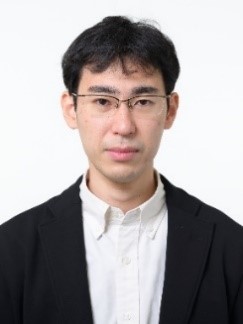
IKE, Yuichi
Degree: PhD (Mathematical Science) (The University of Tokyo)
Research interests: Topological Data Analysis, Microlocal Sheaf Theory
I am working on topological data analysis and sheaf theory. These may seem quite different, as applied and pure mathematics, respectively, but their relationship has been actively studied recently.
(1) Topological Data Analysis (Persistent Homology)
When we look at two point clouds in Figure 1, we humans can distinguish them by the presence of a hole. Topological data analysis is a method for extracting such “rough shapes” of data and making computers handle such information. In mathematics, the “rough shape” of a space is studied in the field of topology, and the number of holes can be extracted by a tool called homology. Upon this framework, the simplest idea to study the shape of a point cloud is to consider the union of discs of a certain radius r centered at each point and look at their homology (Figure 2).
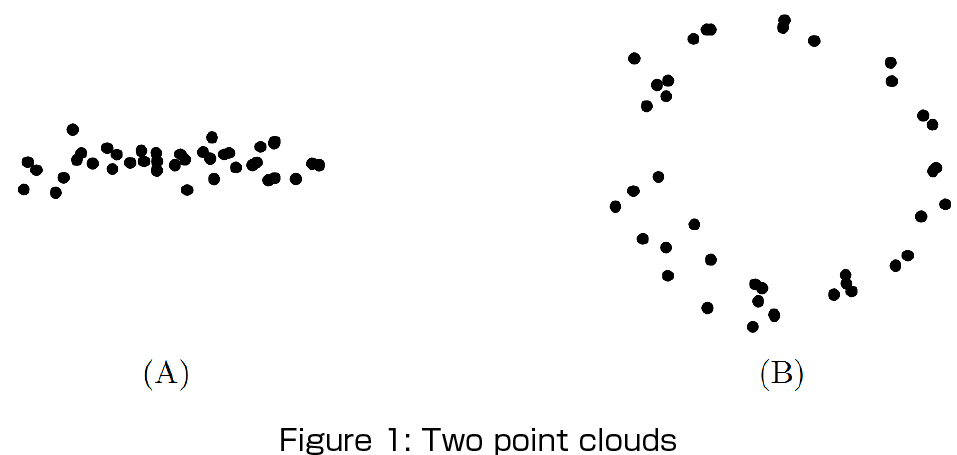
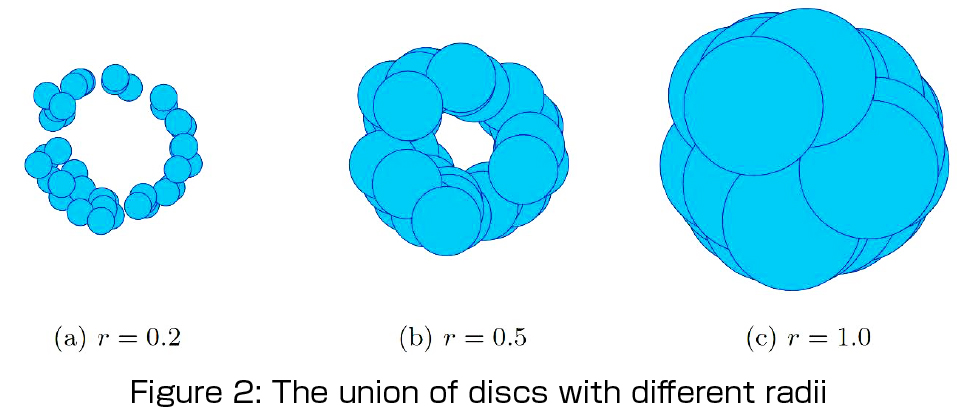
However, with this method, depending on the radius of the discs, we may see only small holes that come from noises (Figure 2(a)) or no holes at all (Figure 2(c)). Thus, the extracted shape is highly dependent on the choice of the radius r, and it is generally difficult to determine an appropriate radius r from a given dataset. Here, we give up on setting a single radius r and instead consider how the “shape” of the point cloud, especially the homology, changes when we move the radius r. By looking at the information on how long the holes persist with respect to r, we can distinguish between large holes and noises. This method is called persistent homology.
The features extracted from data in the above way with persistent homology, which represent the “rough shapes” of the data, can be used as the input for machine learning and sometimes are useful for various tasks. I am interested in applying persistent homology to neural networks to examine the state of the networks and in using persistent homology to train the networks.
(2) Sheaf Theory and Persistent Homology
In addition to the above research, I am also studying the connection between sheaf theory and persistent homology. Sheaves are mathematical objects that are useful in algebraic geometry and topology. In recent years, there have been appeared some attempts to understand persistent homology from the viewpoint of sheaves.
I am interested in the sheaf-theoretic interpretation of the distance for persistent homology and in using this understanding for topological data analysis or other areas in mathematics. Recently, I investigated the relationship between the distance for sheaves and the distance between zigzag persistence, as well as the relationship to energy in symplectic geometry.
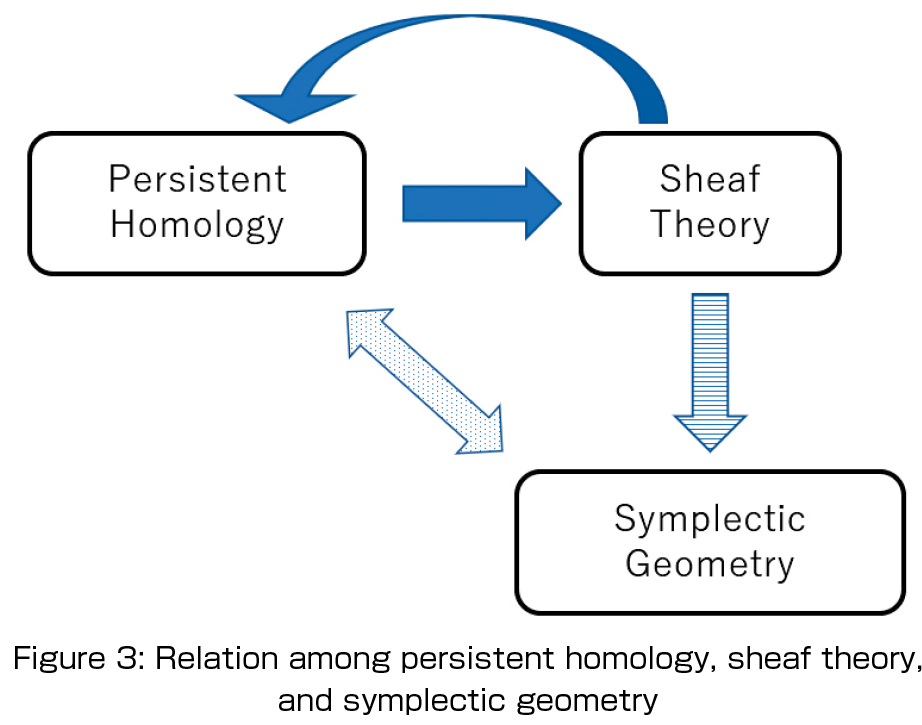
I hope my research in the intersection of applied and pure mathematics will cause an exciting exchange across these areas.