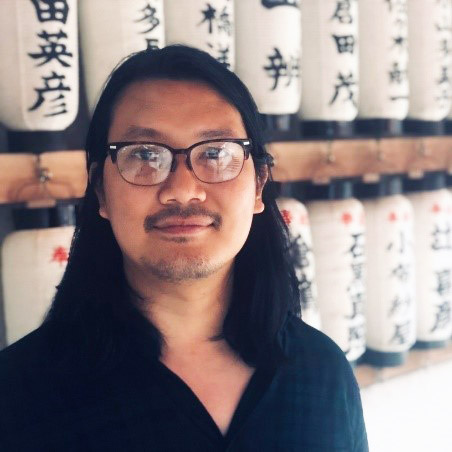
(理論面の)対象 | 数理統計学、確率論、統計学習理論、機械学習、最適化 |
(理論面の)目標 | 統計的アルゴリズムや機械学習アルゴリズムの数学的基礎を発展させ、応用研究におけるベストプラクティスや手続き上の推奨事項に対する洞察を提供する理論的保証を導き出す。 |
ここまでの成果/重要な発見 | 時系列データや空間依存データのクラスタリングを含むクラスタリングの新しいアルゴリズムを開発。大規模仮説検定における偽陽性率を制御する新しい方法を開発した。有限混合モデルを用いた尤度関数フリーベイズ推論のための新しいアルゴリズムを提案し、有限混合モデルと潜在変数モデルを推定するための確率的アルゴリズムを導入した。混合モデルおよび関連する関数構造の表現能力に関する証明を提供。統計的学習課題における一般的なモデル選択のための新しい基礎を確立した。 |
これからの目標/現在取り組んでいる目標 | 混合モデルと混合専門家モデルの性能と機能を評価するための定量的近似定理を確立する。不規則でノンパラメトリックな漸近的シナリオに対する新しい仮説検定の開発 モデル選択ツールを用いた原理的モデル平均化のための新しい手法を導入する。近似理論、学習理論、最新のニューラルネットワークベースのAIアルゴリズム間の相互作用を調査する。 |
応用上の成果/目標 | 私の研究で開発された手法は広く応用できる。例えば、歯科学、漁業・農業、神経画像、神経心理学、薬理学、分子生物学、行動経済学、安全工学などの分野で応用されている。 |
さらなる発展の可能性・方向性 | ニューラルネットワークシステムを理解するための新しいフレームワークの開発。強力な数学的保証のあるニューラルネットワークの推定と学習のための新しいアルゴリズムの構築。異なるモデルの長所を組み合わせて首尾一貫した統計的構成を生成する手法の提案。モデルの誤仕様化に対してロバストで、最小限の仮定で行う検定と推論のための新しい統計手法の開発。 |
Dr. Nguyen is trained as a computational statistician, specializing in the modeling of heterogeneous processes. In this realm, his approach is to model multi-population data using finite mixtures of probability measures and regression models. Each mixture component can be considered as a sub-population of an overall super population characterized by the mixture model. These mixture models have far-reaching applications, and Dr. Nguyen has had the privilege of collaborating with professionals from various fields, including neural and cell biologists, satellite and brain imaging experts, quantum physicists, fisheries scientists, and economists, among others. These professionals have required expertise in modeling their multimodal data, which ranges from zebrafish and human brains to multispectral satellite images and fisheries population stock data.
In his work toward better modeling and estimation of complex data-generating processes, Dr. Nguyen has also developed expertise in the theory of optimization for statistical and probabilistic methods. His focus in this area is on the so-called Majorization-Minimization family of algorithms, a diverse method for constructing optimization algorithms that unify the theory of many modern approaches, such as proximal and gradient descent, convex-concave procedures, expectation-maximization, and many others. His current focus in this area is on developing stochastic and mini-batch variants for the optimization of random processes, such as sample averages and other statistical procedures, in situations where data may not be available all at once. This is particularly prevalent when data are very large, require more storage than is available, or arrive in a stream, such as data acquired online or in a monitoring process.
Through his work with and use of mixture model constructions, Dr. Nguyen has developed novel results towards the understanding of such objects and has written broadly on the topic of their approximation theoretic properties. To this end, he has provided analyses of the limitations and capabilities of mixture models and mixture regressions with respect to the potential shapes and forms that they can take and how broadly applicable they are to the general analysis of statistical processes. Furthermore, the application of diverse classes of mixture models and other techniques has led Dr. Nguyen to also study the theory of model selection. Here, he has developed new theoretical procedures and provided new insights into currently used procedures for choosing between competing statistical models in a systematic and principled way.
In his current research, Dr. Nguyen has also explored the analysis of data from a Bayesian perspective, wherein one wishes to integrate knowledge from observations together with objective and subjective prior knowledge of the underlying data-generating process. His interest in Bayesian analysis lies within the realm of approximate Bayesian inference, where the data-generating process is intractable and requires accurate approximation.
キーワード | Mathematical Statistics; Statistical Computing; Statistical Learning; Bayesian Statistics; Signal Processing; Stochastic Programming; Optimization Theory |
---|---|
部門 | 産業数理統計研究部門,オーストラリア分室 (兼任) |
リンク | Homepage |