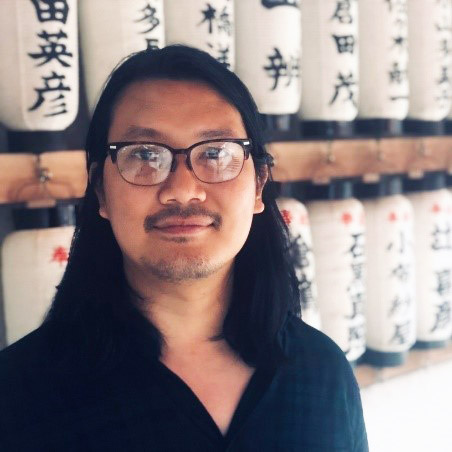
Dr. Nguyen is trained as a computational statistician, specializing in the modeling of heterogeneous processes. In this realm, his approach is to model multi-population data using finite mixtures of probability measures and regression models. Each mixture component can be considered as a sub-population of an overall super population characterized by the mixture model. These mixture models have far-reaching applications, and Dr. Nguyen has had the privilege of collaborating with professionals from various fields, including neural and cell biologists, satellite and brain imaging experts, quantum physicists, fisheries scientists, and economists, among others. These professionals have required expertise in modeling their multimodal data, which ranges from zebrafish and human brains to multispectral satellite images and fisheries population stock data.
In his work toward better modeling and estimation of complex data-generating processes, Dr. Nguyen has also developed expertise in the theory of optimization for statistical and probabilistic methods. His focus in this area is on the so-called Majorization-Minimization family of algorithms, a diverse method for constructing optimization algorithms that unify the theory of many modern approaches, such as proximal and gradient descent, convex-concave procedures, expectation-maximization, and many others. His current focus in this area is on developing stochastic and mini-batch variants for the optimization of random processes, such as sample averages and other statistical procedures, in situations where data may not be available all at once. This is particularly prevalent when data are very large, require more storage than is available, or arrive in a stream, such as data acquired online or in a monitoring process.
Through his work with and use of mixture model constructions, Dr. Nguyen has developed novel results towards the understanding of such objects and has written broadly on the topic of their approximation theoretic properties. To this end, he has provided analyses of the limitations and capabilities of mixture models and mixture regressions with respect to the potential shapes and forms that they can take and how broadly applicable they are to the general analysis of statistical processes. Furthermore, the application of diverse classes of mixture models and other techniques has led Dr. Nguyen to also study the theory of model selection. Here, he has developed new theoretical procedures and provided new insights into currently used procedures for choosing between competing statistical models in a systematic and principled way.
In his current research, Dr. Nguyen has also explored the analysis of data from a Bayesian perspective, wherein one wishes to integrate knowledge from observations together with objective and subjective prior knowledge of the underlying data-generating process. His interest in Bayesian analysis lies within the realm of approximate Bayesian inference, where the data-generating process is intractable and requires accurate approximation.
キーワード | Mathematical Statistics; Statistical Computing; Statistical Learning; Bayesian Statistics; Signal Processing; Stochastic Programming; Optimization Theory |
---|---|
部門 | 産業数理統計研究部門,オーストラリア分室 (兼任) |
リンク | Homepage |